Let’s get ready to rumble! This is a machine translation (MT) battle royal between DeepL Translator, Google Translate, and Microsoft Translator. I thought it might be interesting to select a popular media article, which uses some slang and pit these three neural machine translation engines against each other.
Let’s meet our competitors.
–
The Competitors
DeepL
DeepL Translator was introduced by DeepL, previously Linguee, in August of 2017. With a catchy name taken from Deep Learning, early reviews of DeepL’s performance said this underdog raised the bar for the field.
Google Translate
Google may have discontinued the website widget for new sites in 2019, but the tech giant is still strong in real-time document, text, and website translation to this day.
Microsoft Translator
Also known as Bing Translator, and part of Azure Cognitive Services, Microsoft Translator offers text and speech translation from another tech giant which brings the power of Microsoft into the ring.
–
The Article
The article we chose appeared in the online news source, The Morning Brew. It was chosen due to its popularity in current events. The article covered the effort of the Retail, Wholesale, and Department Store Union (RWDSU) to unionize the employees at an Amazon facility in Alabama. The union contends that employees at the facility were being treated unfairly. The complaints centered around a lack of communication between employees and management. The union is facing an uphill battle against Amazon.
The language used is relatively simple but there certainly is some nuance to certain parts of the story. Some examples of nuanced language from the article are:
- Friends in high places
- Rippled across the e-commerce industry
- The generic term officials
We ran the story through each of the three MT engines in German and Japanese.
–
The Judges
Gerhard Preisser is an accomplished German translator working with some of the best translation services firms for over 26 years. He previously taught German at Penn State, Georgetown University, and the Catholic University of America, and English at City Colleges of Chicago.
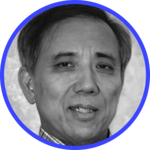
Susumu Fukase is an accomplished Japanese translator with over 40 years of experience and works with some of the finest translation agencies all over the world.
–
The Main Event
Let the battle begin. Seriously, let’s take a look at how the tools did on the translation effort. In reading the English, you will see some nuanced language and some slang. This is typically where this type of translation tool will have issues.
A few general observations about both languages:
- None of our 3 contestants got the headline right, and each one of them had trouble understanding the nuance behind “It may not end today”.
- None of them was able to accurately translate “organizers” and “organizing” in the context of unions.
- Each one of them mistranslated “officials” as “government employees.”
- Each one of them had trouble with “With about half the ballots remaining…”, resulting in mistranslations.
- In many spots the translation was too literal and too mechanical, stylistic changes in translation would have improved the resulting translations
Generally, and this goes for all three translations, there is a significant lack of what I’d call stylistic consistency. Some sentences are handled quite well and appropriately, given the fact that this is essentially a newspaper story, but then, suddenly, there’s a noticeable, even startling change in tone/register and in the case of Japanese, a change to using Katakana where it wouldn’t be appropriate. Had our team been asked to give this a thorough edit to make it publication-ready, a complete rewrite would be suggested.
–
“Had this been the work of a new human translator… I would have suggested that you go look again.”
–
On the other hand, the translations do manage to tell the intended story—not in any elegant or captivating fashion, but in a way that allows the reader to come away with a general understanding of the facts (of course this is after getting past the headline and understanding the whole union angle of what happened with Amazon in Alabama). They do provide a helpful overview and give the reader an idea of what the article is all about.
On a positive note, DeepL did a nice job handling some colloquial phrases in Japanese like “grew fed up with” and “friends in high places”.
When our Judges were asked to rank our three contestants, they said that DeepL did the best job overall, followed by Google. Microsoft comes in last, mostly because it invented new words and made a number of mistakes that distort the intended meaning.
Gerhard had a particularly interesting comment to offer. “Had this been the work of a new human translator (who wished to be hired), and this goes for all 3 solutions and had you asked me to assess his/her qualifications, I would have suggested that you go look again.”
Susumu weighed in and suggested the following. “In terms of translation, MT should be considered a good and tireless hard-working co-worker that can provide useful information and reference when it comes to the translation. However, we need a well-qualified human translator to produce a high-quality translation. It is up to linguists as to whether we want to accept a translation by using MT.”
–
The Winner for Best MT Translation
The final bell has rung, and the apparent winner is…. DeepL! Overall DeepL seemed to manage nuance and natural language in the best way possible.
All three platforms had their issues, and DeepL might not be in the same weight class as the other contenders, but it definitely provides acceptable first pass translation for non-customer facing materials.
Machine translation can be a part of a translation system, but only when utilized for the right use case and situation. We would encourage you to talk about the risks and how to properly adjust workflows to include machine translation in your toolset.